Data Science Training in Bangalore
Recent advancement in Artificial intelligence and machine learning has led to a lot of searches for data science training courses in Bangalore. At Programink Bangalore, we provide an end-to-end solution for data science training covering real-life implementation knowledge, certificate and placement. We truly believe in delivering quality content which are up to the mark with the current market standards with the Chinese philosophy that "There are no bad students, only bad teachers". So if you have willingness to learn and an unapologetic attitude to achieve your own commitments then Programink is the right choice for you.
Data Science Course Highlights
- 50 hours of instructor-led training
- 16 hours of project work
- 6 hours of assessments
- 1 year of learning support
- Certificate
- Job assistance
100% money-back guarantee:
Our Data Science training course in Bangalore comes with a 100% money-back guarantee. Any student can cancel registration with-in the first week of course commencement and get a full refund of the tuition fee.
Additional Benefits: The need for certified Data Engineers is increasing every year. There is a positive growth in the job opportunities for professionals trained in AWS.
Data Science Course Curriculum
We have the most detailed course curriculum for Data Science training with complete coverage of all important topics and live industry projects.
Eligibility: The Data Science certification training in Bangalore is ideal for both beginners and working professionals. Anyone who wants to data analytics, statistics, data modeling, data visualization and machine learning operations can opt for this Data Science training in Bangalore.
Pre-requisites: There are no strict prerequisites for this AWS training course. Simply a basic understanding of computing and python programming is recommended.
- 1. Descriptive Statistics
- 2. Measure of central tendency
- 3. Distributions
- 4. Hypothesis testing
- 5. Inferential statistics
- 6. Regression Analysis
- 1. SQL Basics
- 2. Data types and constraints
- 3. CRUD Operations
- 4. SQL Joins
- 5. SQL commands and clauses
- 6. Data analytics with Excel
- 1. NumPy
- 2. Pandas
- 3. Data Assembly
- 4. Missing Data
- 5. Data Munging
- 6. Pandas advance operations
- 1. matplotlib
- 2. seaborn
- 3. Exploratory data analysis
- 4. Business Intelligence
- 1. Regression and Classification Models
- 2. Linear Regression Mode
- 3. Logistic Regression Model
- 4. Evaluation Metrics for Classification model
- 5. K Nearest Neighbors Model
- 6. Decision Tree Model
- 7. Random Forest Model
- 8. K Means and Hierarchical Clustering
- 9. Principal Component Analysis (PCA)
- 10. Support Vector Machine (SVM)
- 11. Feature Engineering
- 12. Time Series Forecasting
- 1. Deep Neural Network
- 2. TensorFlow
- 3. Computer vision
- 4. Natural language processing (NLP)
- 1. Deployment of ML Model in the Cloud
- 2. AWS Cloud for Data Science
- 3. Azure for Data Science
Data Science Course Projects
Project 1: Automate EC2 instance creation
Create and configure EC2 with Python and Shell scripting.
Project 2: AWS Lambda and Load-balancer Configurations
Launch two or more web servers and configure a load-balancer with auto-scaling.
Project 3: E-commerce Solution Architecture
Take multiple architectural decision for an e-commerce application.
Frequently asked questions for Data Science training course in Bangalore
Data science is a field of computer science where we deal with data gathering, analyzing and solving a problem with the help of data and algorithms.
Data science is a field of computer science where we deal with data gathering, analyzing and solving a problem with the help of data and algorithms.
Programink gives you the promise to teach in-line with Data Science Certification Exam Guide and the training will be delivered by an Amazon certified trainer. Apart from that, the training path is very engaging and the practice tests are at par with the actual certification exam. Take a demo class to find more reasons to believe, we provide the best Data Science training in Bangalore.
Programink gives you the promise to teach in-line with Data Science Certification Exam Guide and the training will be delivered by an Amazon certified trainer. Apart from that, the training path is very engaging and the practice tests are at par with the actual certification exam. Take a demo class to find more reasons to believe, we provide the best Data Science training in Bangalore.
Gathering, analyzing and solving a problem with the help of data and algorithms is the crude definition of data science. Just like any other branch of science, Data Science heavily consists of experiments, research and ground breaking findings. It is the intersection between Mathematics & Computer Science and given the IT boom the application of data science in today’s tech heavy world is endless. You can be sure that some of the major challenging problems in e-commerce, pharmaceuticals, banking, logistics, medicine, astrophysics etc. are being solved with the help of data science.
A Data Scientist is a qualitative thinker and have mastered at least one programming language for crunching the data and knows one or more languages to mine the data out of the system. For a data scientist the core skill is his/her analytical thinking and the way he approaches a problem. He could break a complex problem in smaller chunks and can look at a single problem while shifting the paradigm so that no aspect of the problem remains untouched. And finally, a data scientist is skilled in devising strategies from the gathered observation while justifying those strategies with data and communicate them in ways that can be easily digested. Just like a great Storyteller the data scientist projects his work like a movie which is engaging at multiple levels and delivers a message which is understood and applied by the stake holder.
Gathering, analyzing and solving a problem with the help of data and algorithms is the crude definition of data science. Just like any other branch of science, Data Science heavily consists of experiments, research and ground breaking findings. It is the intersection between Mathematics & Computer Science and given the IT boom the application of data science in today’s tech heavy world is endless. You can be sure that some of the major challenging problems in e-commerce, pharmaceuticals, banking, logistics, medicine, astrophysics etc. are being solved with the help of data science.
A Data Scientist is a qualitative thinker and have mastered at least one programming language for crunching the data and knows one or more languages to mine the data out of the system. For a data scientist the core skill is his/her analytical thinking and the way he approaches a problem. He could break a complex problem in smaller chunks and can look at a single problem while shifting the paradigm so that no aspect of the problem remains untouched. And finally, a data scientist is skilled in devising strategies from the gathered observation while justifying those strategies with data and communicate them in ways that can be easily digested. Just like a great Storyteller the data scientist projects his work like a movie which is engaging at multiple levels and delivers a message which is understood and applied by the stake holder.
Although a certification adds value to your resume but some good capstone projects and an in-depth knowledge of the subject trumps any certification. So it would be fair to say that having certification is not a game changer if you cannot back that up with a knowledge and hands on experience. A recruiter would rather look for projects and experience to assess your skills as a data scientist and not for a certificate whose credibility on the rigorousness and content are questionable. It just does not make any sense for a recruiter to blindly believe that a certification can bypass knowledge that need to weigh before coming to a conclusion.
If you are a fresher an in depth knowledge of the projects that you have put in your resume and the link to your GitHub account should suffice the need, and if you are an experience personal then in addition to aforementioned requirements you would be assessed on your domain and industry experience. If you are comfortable with your projects and have confidence in your skill set you would always have a competitive advantage over any certificate holder.
Although a certification adds value to your resume but some good capstone projects and an in-depth knowledge of the subject trumps any certification. So it would be fair to say that having certification is not a game changer if you cannot back that up with a knowledge and hands on experience. A recruiter would rather look for projects and experience to assess your skills as a data scientist and not for a certificate whose credibility on the rigorousness and content are questionable. It just does not make any sense for a recruiter to blindly believe that a certification can bypass knowledge that need to weigh before coming to a conclusion.
If you are a fresher an in depth knowledge of the projects that you have put in your resume and the link to your GitHub account should suffice the need, and if you are an experience personal then in addition to aforementioned requirements you would be assessed on your domain and industry experience. If you are comfortable with your projects and have confidence in your skill set you would always have a competitive advantage over any certificate holder.
For a fresher, even if you start today you would need 3-4 months of dedicated learning to land an entry level job as Junior/Associate Data Scientist. If you put in more hours to gain the domain and industry experience you could even convert a Data scientist role opportunity directly. But for fresher it generally tougher because this position always demands an extraordinary combination of computer science, math, industry exposure, domain knowledge and corporate experience. All of these, for a fresher, is tough to bring on to the table for obvious reasons. But nevertheless, if you believe in yourself and are willing to put the desired work against your commitments then you will excel and would defiantly bypass all the social norms and at Programink we commit to walk this difficult path along with you so we can help you reach your career goals.
For an experienced personal it is comparatively easier as out of the required prerequisite the experience of the personal brings in the domain knowledge, industry exposure and the corporate experience by default. Rest of the skills like mathematics, computer science and hands on projects could be gained over 4-5 months of rigorous training in the subject while working on some complex problems which would help reshape your profile. Even if you’re experienced in domains which does not directly translates to data science or information technology we at programink guarantee you to guide you in path which would help you make this shift in career a cake walk. So sum things up for an experienced professional, apart from the evident advantages you would get from your work experience if you could add the required Mathematics and projects to your arsenal than you would be ready to enter the race.
For a fresher, even if you start today you would need 3-4 months of dedicated learning to land an entry level job as Junior/Associate Data Scientist. If you put in more hours to gain the domain and industry experience you could even convert a Data scientist role opportunity directly. But for fresher it generally tougher because this position always demands an extraordinary combination of computer science, math, industry exposure, domain knowledge and corporate experience. All of these, for a fresher, is tough to bring on to the table for obvious reasons. But nevertheless, if you believe in yourself and are willing to put the desired work against your commitments then you will excel and would defiantly bypass all the social norms and at Programink we commit to walk this difficult path along with you so we can help you reach your career goals.
For an experienced personal it is comparatively easier as out of the required prerequisite the experience of the personal brings in the domain knowledge, industry exposure and the corporate experience by default. Rest of the skills like mathematics, computer science and hands on projects could be gained over 4-5 months of rigorous training in the subject while working on some complex problems which would help reshape your profile. Even if you’re experienced in domains which does not directly translates to data science or information technology we at programink guarantee you to guide you in path which would help you make this shift in career a cake walk. So sum things up for an experienced professional, apart from the evident advantages you would get from your work experience if you could add the required Mathematics and projects to your arsenal than you would be ready to enter the race.
The fact that 90% of all the data available today was generated in the past 3 years vouch for the insight that human resources needed to handle and analyze the data is only going to grow in the coming decade. The cost of storing the data and cost of computing & crunching the data has fallen dramatically there by empowering even SMEs along with giant MNCs to get insights with help of data scientists from the massive data warehouses which were previously untouched.
Approximately 1,00,000 analytics jobs are stilled required to be filled in India which is 45% more than last year (2019). The fact that India only contributes to 6% of the Data Science job openings worldwide & the growth of the demand of data scientists worldwide was higher than India tells us that Indian market is just warming up to get into the world wide data science race. Further, companies like Accenture, Amazon, KPMG, Honeywell, Wells Fargo, Ernst & Young, Hexaware Technologies, Dell International, eClerx Services & Deloitte including their offices in India were the leading organisation with most number of Data science and analytics opening. To all the above mentioned facts if we add the insights that an analytics position on an average remains open for only 45 days we can easily infer that the world is increasingly demanding more data muscle to push itself in this age of information.
The fact that 90% of all the data available today was generated in the past 3 years vouch for the insight that human resources needed to handle and analyze the data is only going to grow in the coming decade. The cost of storing the data and cost of computing & crunching the data has fallen dramatically there by empowering even SMEs along with giant MNCs to get insights with help of data scientists from the massive data warehouses which were previously untouched.
Approximately 1,00,000 analytics jobs are stilled required to be filled in India which is 45% more than last year (2019). The fact that India only contributes to 6% of the Data Science job openings worldwide & the growth of the demand of data scientists worldwide was higher than India tells us that Indian market is just warming up to get into the world wide data science race. Further, companies like Accenture, Amazon, KPMG, Honeywell, Wells Fargo, Ernst & Young, Hexaware Technologies, Dell International, eClerx Services & Deloitte including their offices in India were the leading organisation with most number of Data science and analytics opening. To all the above mentioned facts if we add the insights that an analytics position on an average remains open for only 45 days we can easily infer that the world is increasingly demanding more data muscle to push itself in this age of information.
Programink offers a Data Science course in Bangalore which is the most comprehensive Data Science course in the market. It covers the complete Data Science life-cycle concepts from Data Collection, Data Extraction, Data Cleansing, Data Exploration, Data Transformation, Feature Engineering, Data Integration, Data Mining, building Prediction models, Data Visualization and deploying the solution to the customer.
This Data Science training covers a wide range of skills and tools, including statistical analysis, text mining, regression modeling, hypothesis testing, predictive analytics, machine learning, deep learning, neural networks, natural language processing, predictive modeling, R Studio, Tableau, Spark, Hadoop, and programming languages like Python and R. With over 400 participants placed in various multinational companies like E&Y, Panasonic, Accenture, VMWare, Infosys, IBM, etc., Programink is regarded as the best Data Science training institute in Bangalore. The program offers services ranging from training to placement. Programink is regarded as the best in the industry and offers the best data science training in Bangalore.
Programink offers a Data Science course in Bangalore which is the most comprehensive Data Science course in the market. It covers the complete Data Science life-cycle concepts from Data Collection, Data Extraction, Data Cleansing, Data Exploration, Data Transformation, Feature Engineering, Data Integration, Data Mining, building Prediction models, Data Visualization and deploying the solution to the customer. This Data Science training covers a wide range of skills and tools, including statistical analysis, text mining, regression modeling, hypothesis testing, predictive analytics, machine learning, deep learning, neural networks, natural language processing, predictive modeling, R Studio, Tableau, Spark, Hadoop, and programming languages like Python and R. With over 400 participants placed in various multinational companies like E&Y, Panasonic, Accenture, VMWare, Infosys, IBM, etc., Programink is regarded as the best Data Science training institute in Bangalore. The program offers services ranging from training to placement. Programink is regarded as the best in the industry and offers the best data science training in Bangalore.
Most institutes are attaching an unworthy certification and charging close to 5,00,000 for the same course that we bring you at 90,000. With some additional discounts you many avail it at 63,000.
Most institutes are attaching an unworthy certification and charging close to 5,00,000 for the same course that we bring you at 90,000. With some additional discounts you many avail it at 63,000.
Yes. It is quite possible to master all the key skills in Data Science within 3 months. But if you completely lag the understanding of programming and statistics, we would advice you to up-skill that first.
Yes. It is quite possible to master all the key skills in Data Science within 3 months. But if you completely lag the understanding of programming and statistics, we would advice you to up-skill that first.
Give a chance and you will find that we have a training path that starts at your current understanding of the subject and teaches everything else that the industry expects of you.
Give a chance and you will find that we have a training path that starts at your current understanding of the subject and teaches everything else that the industry expects of you.
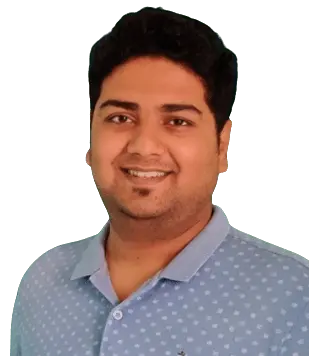
My recent corporate clients
Please find a list of some top corporate clients I have trainer on this technology.
- Deloitte
- Walmart
- Oracle
- Cisco
- Brillio
- PayU
- Capgemini
- Cognizant
- Innovaccer
- Sony
- Siemens
- ANZ
- WSP
- Society General
- Optum
- Carrier
- Harman
- Ellucian
- Bank of America
- Pepsico
- Konsberg
- Infrrd
- Tata Technologies
- Collins Aerospace
- Morgan Stanley
Data Science Course Ratings & Reviews
Data Science training locations
Data Science Training in Bangalore
Data Science Course in Bangalore
Data Science Training in BTM Layout
Data Science Training in Jayanagar
Data Science Training in Banashankari
Data Science Course Fees in Bangalore
Data Science Training in Electronic City
Data Science Training near Silk board
Data Science Training in Marathahalli
Data Science Training in Bellandur
Data Science Training in Sarjapur Road
Data Science Training in HSR Layout
Data Science Training in Koramangala